Once you've mastered the basics of prompt engineering, it's time to take your AI-generated images to the next level. This guide explores advanced techniques for optimizing your AI artwork through post-processing, parameter fine-tuning, and workflow enhancements.
A reference landscape showing the level of detail and composition possible with advanced optimization techniques
Understanding Image Quality Factors
Several factors influence the quality of AI-generated images:
1. Resolution and Detail
Higher resolution doesn't always mean better images. Each AI model has an optimal resolution range where it performs best. For many models, this is around 768x768 or 1024x1024 pixels.
Adding parameters like "high resolution," "detailed," or "8k" can improve perceived detail, but understanding your specific model's capabilities is crucial.
With newer models like Stable Diffusion 2.0 and beyond, the resolution capabilities have expanded, supporting up to 2048×2048 with better coherence at higher resolutions compared to earlier versions.
2. Coherence and Composition
AI models sometimes struggle with complex compositions or unusual perspectives. Creating coherent images often requires:
- Clear compositional guidance (foreground, middleground, background)
- Consistent perspective cues
- Balanced element distribution
- Specific camera position descriptors (e.g., "bird's eye view," "low angle shot")
3. Consistency of Style
Maintaining a consistent style throughout an image can be challenging. Using specific artist references or style descriptors helps, but be aware that mixing too many style references may create confused results.
For the most consistent results, consider limiting yourself to 1-3 style references and ensuring they complement each other (e.g., combining "art nouveau" and "watercolor" works better than "cubism" and "hyperrealism").
Example of consistent style application: note how lighting, atmosphere and perspective work together harmoniously
Advanced Parameter Optimization
Sampling Methods
Different sampling methods produce varying results:
- Euler a: Good for artistic, painterly images
- DPM++ 2M Karras: Excellent for photorealistic details
- DDIM: Creates more predictable, stable outputs
- Heun: Balances detail and creativity, good for portrait work
- DPM++ SDE Karras: Produces high-quality details with natural variations
Experiment with various sampling methods to find what works best for your specific visual goals.
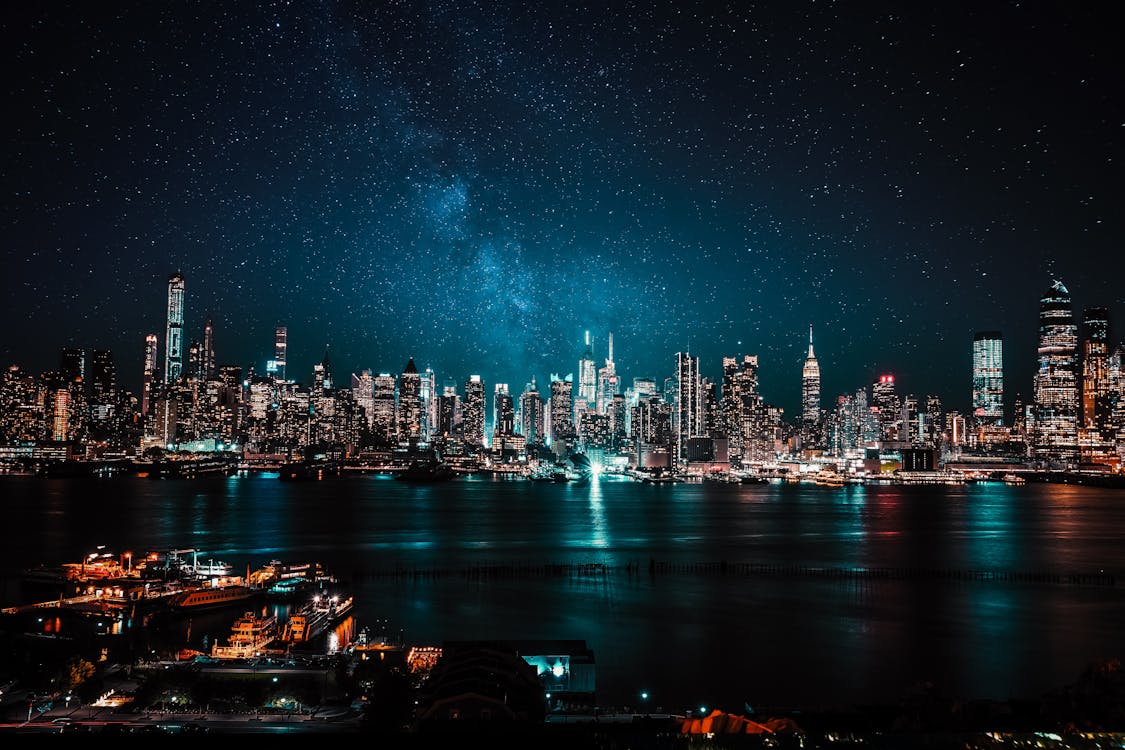
The level of detail and texture in this scene demonstrates what can be achieved with optimal sampling method selection
CFG Scale (Classifier Free Guidance)
This parameter controls how closely the AI follows your prompt:
- Low values (5-7): More creative freedom, potentially more aesthetic but less prompt-adherent
- Medium values (7-9): Balanced approach for most use cases
- High values (10+): Strict prompt adherence, potentially over-saturated or contrasty images
For maximum realism in photographic styles, values between 7-8 often produce the most balanced results without artifact exaggeration.
Steps and Seed Values
The number of sampling steps affects detail refinement:
- 20-30 steps are sufficient for most images
- 35-50 steps can help with complex, detailed scenes
- Diminishing returns typically occur beyond 50 steps
Saving seed values of successful generations allows you to create variations while maintaining the core composition.
Pro tip: Create a "seed library" of compositions you like, organized by category (landscapes, portraits, abstract). This can save significant time when developing a series of related images.
The Power of Negative Prompts
Negative prompts tell the AI what you don't want in your image, often improving quality by eliminating common artifacts:
- Basic quality issues: "blurry, low quality, pixelated, ugly, distorted"
- Anatomy problems: "deformed hands, extra fingers, missing limbs, extra limbs, bad anatomy"
- Compositional issues: "cropped image, bad framing, out of frame, poorly composed"
- Style avoidance: "cartoon, anime, 3d render, clipart" (when aiming for photorealism)
Be careful not to over-constrain your image with too many negative prompts, as this can limit the AI's creative range. Start with negative prompts addressing common issues, then add specific negatives based on unwanted elements in your initial generations.
Refined image created using negative prompts to eliminate common AI generation artifacts
Workflow Optimization Strategies
The Iterative Refinement Method
Rather than trying to get the perfect image in one attempt, use this multi-stage approach:
- Composition Stage: Generate a basic version focusing on layout and composition
- Detail Enhancement: Use img2img with the first result, adding more specific details
- Style Refinement: Adjust artistic style parameters while maintaining structure
- Final Touches: Fine-tune with subtle adjustments to lighting, color, or specific elements
This approach is particularly effective for complex scenes or when you have a clear vision but are struggling to achieve it in a single generation.
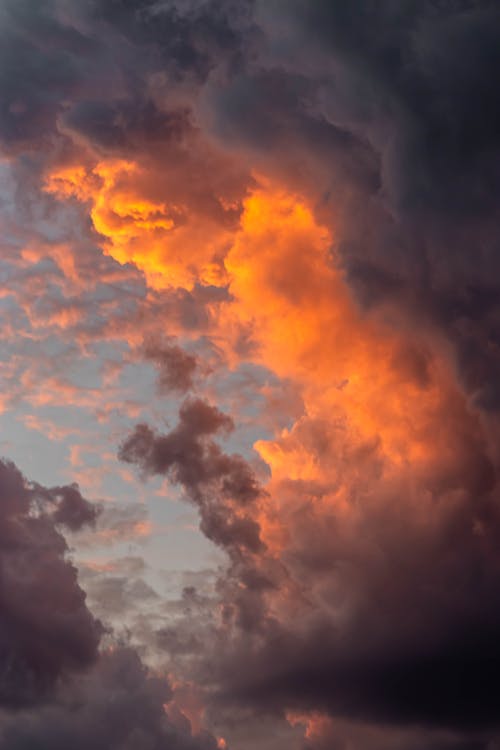
A complex scene like this is best approached through iterative refinement rather than attempting to generate it in one step
ControlNet Techniques
ControlNet represents a major advancement in AI image generation, giving you precise control over specific aspects of your image:
- Pose Control: Apply specific body poses from reference images
- Depth Maps: Maintain the spatial relationships and composition of a reference
- Canny Edge: Preserve structural elements while changing style and content
- Scribble: Transform rough sketches into detailed images
- Reference-Only: Preserve specific elements while completely transforming others
For best results with ControlNet, start with a clear reference image or precise control map. The quality of your control input directly impacts your results.
Example of how ControlNet can transform a simple sketch into a detailed image while preserving the original composition
Inpainting and Outpainting Techniques
For precise control over specific areas:
- Inpainting: Selectively regenerate problematic areas while preserving the rest
- Region-specific prompts: Apply different text prompts to different regions of your image
- Masked generation: Use transparency masks to control which areas are affected
- Outpainting: Expand your canvas beyond the original boundaries for larger scenes or additional elements
Advanced tip: Create layer-based inpainting workflows by saving intermediate masks. This allows you to "undo" specific changes without starting over completely.
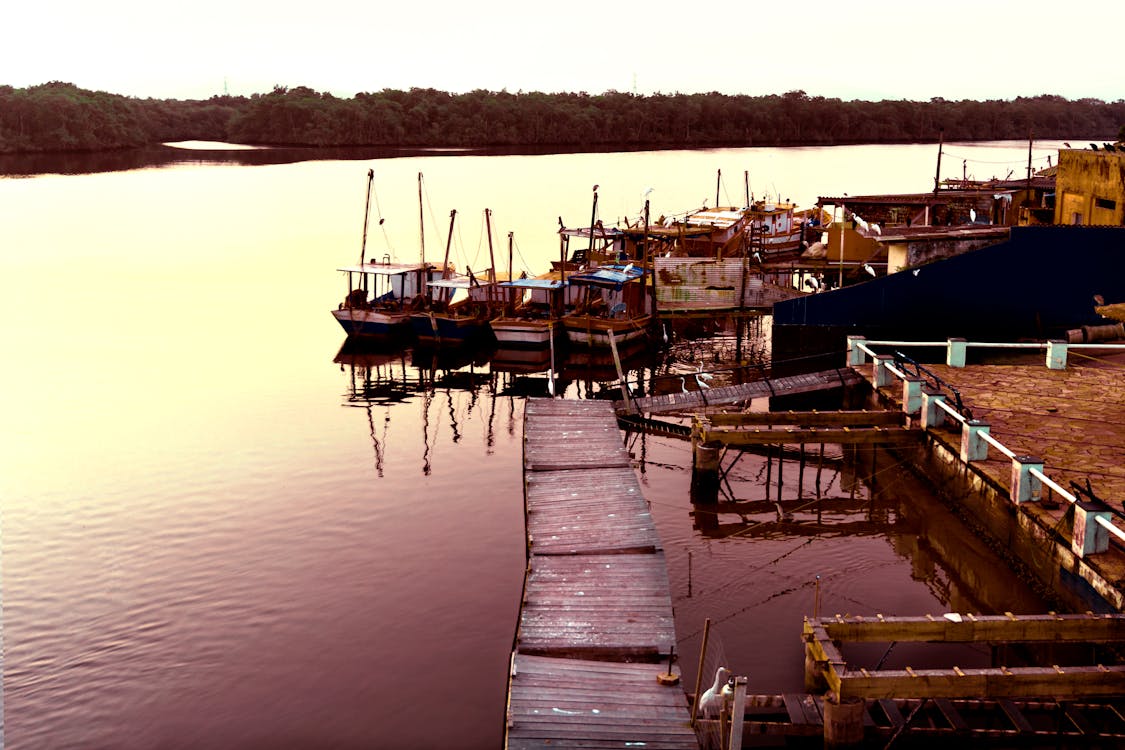
Selective modification through inpainting allows for precise control over specific elements
Prompt Chaining
Create a series of related prompts that build upon each other:
Stage 1: "rough concept sketch of a futuristic city skyline"
Stage 2: "detailed architectural rendering of a futuristic city skyline with flying vehicles, based on previous concept"
Stage 3: "photorealistic cityscape of a futuristic metropolis with flying vehicles, gleaming skyscrapers, golden hour lighting"
This approach helps the AI progressively refine complex ideas, particularly for scenes with multiple elements or detailed environments.
Final stage result of prompt chaining showing a detailed futuristic cityscape
Image-to-Image Pipelines
Advanced workflows often involve a series of transformations:
- Base Generation: Create your initial composition
- Style Transfer: Apply a specific aesthetic while maintaining content
- Detail Enhancement: Focus on specific areas that need improvement
- Artifact Removal: Clean up any generation artifacts or inconsistencies
Keep denoising strength between 0.4-0.7 for img2img to maintain the original structure while allowing for creative development.
When to Use External Software
Consider using dedicated photo editing software when:
- Combining multiple AI generations into a single composition
- Creating consistent series of images with identical color grading
- Requiring precise control over specific elements
- Preparing images for professional printing or publication
- Applying advanced masking that exceeds AI inpainting capabilities
- Needing pixel-perfect precision for commercial work

Professional editing workflows often combine AI-generated elements with manual adjustments for optimal results
Optimizing for Specific Visual Styles
Photorealistic Images
For convincing photorealism:
- Reference real camera models and settings (e.g., "shot on Canon EOS R5, 85mm f/1.2 lens")
- Include subtle imperfections (grain, slight lens distortion, chromatic aberration)
- Pay attention to physically accurate lighting (mention light source, quality, and direction)
- Use terms like "photographic," "35mm film," or "documentary style"
- Add technical descriptors like "photogrammetry," "8K resolution," "RAW format"
Newer models trained on higher-quality images (like Stable Diffusion 2.0+) generally produce better photorealistic results than earlier versions.
Photorealistic rendering with careful attention to lighting, natural imperfections, and physical accuracy
Artistic and Stylized Images
For distinctive artistic styles:
- Reference specific artists or art movements (e.g., "in the style of Monet" or "art nouveau aesthetic")
- Combine complementary styles for unique results ("impressionist technique with fantasy subject matter")
- Specify medium (oil paint, watercolor, digital illustration, block print)
- Include technique descriptions (impasto, crosshatching, glazing techniques)
- Reference era-specific details for historical styles ("1920s art deco poster design")
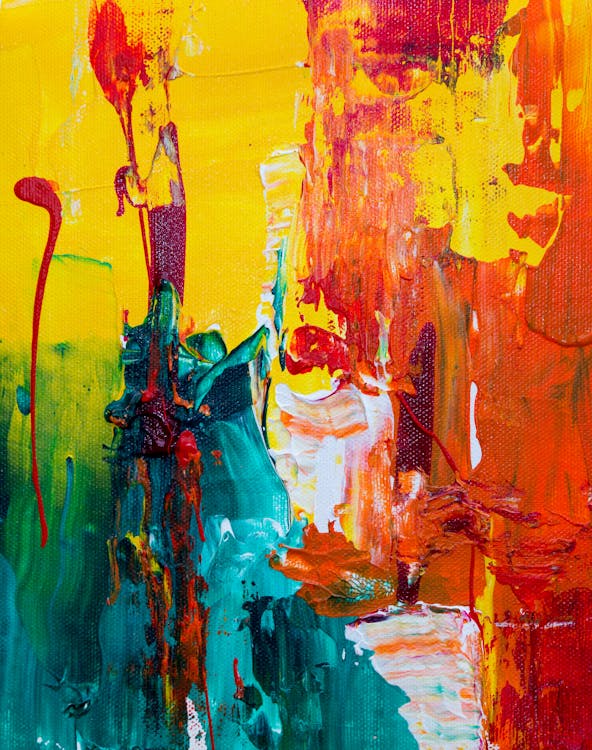
Artistic rendering in an impressionist style demonstrates how specific style references yield distinctive results
Case Study: Transforming a Basic Landscape
Starting Point
Basic prompt: "mountain landscape with lake"
Result: Generic landscape with basic elements but lacking distinction
Starting point: A generic mountain landscape that lacks specific character and atmosphere
Optimization Stage 1: Composition and Setting
Enhanced prompt: "panoramic view of jagged mountain peaks surrounding a crystal clear alpine lake, Swiss Alps"
Improvement: More specific geography and composition guidance
Optimization Stage 2: Atmosphere and Lighting
Enhanced prompt: "panoramic view of jagged mountain peaks surrounding a crystal clear alpine lake in the Swiss Alps, golden hour lighting with long shadows, thin mist rising from the water surface"
Improvement: Added specific lighting condition and atmospheric elements
Optimization stage 2: Note the golden hour lighting and atmospheric mist that create a more compelling scene
Optimization Stage 3: Technical Parameters
Final prompt: "panoramic view of jagged mountain peaks surrounding a crystal clear alpine lake in the Swiss Alps, golden hour lighting with long shadows, thin mist rising from the water surface, captured with wide-angle lens, photorealistic, highly detailed, 8k, professional landscape photography"
Negative prompt: "blurry, oversaturated, cartoon, painting, illustration, cropped, frame, text, watermark, signature, flat lighting, overexposed, underexposed"
Improvement: Added technical specifications, quality parameters, and negative prompts to eliminate common issues
Final result: A professional-quality landscape with optimal technical parameters and detailed rendering
Post-Processing
Final adjustments:
- Enhanced contrast in the mountain areas
- Slightly increased saturation in the golden light areas
- Applied subtle vignetting to focus attention on the center composition
- Removed minor artifacts in cloud formations
- Used selective color adjustment to enhance blues in the lake and sky
- Applied minimal noise reduction to preserve natural texture
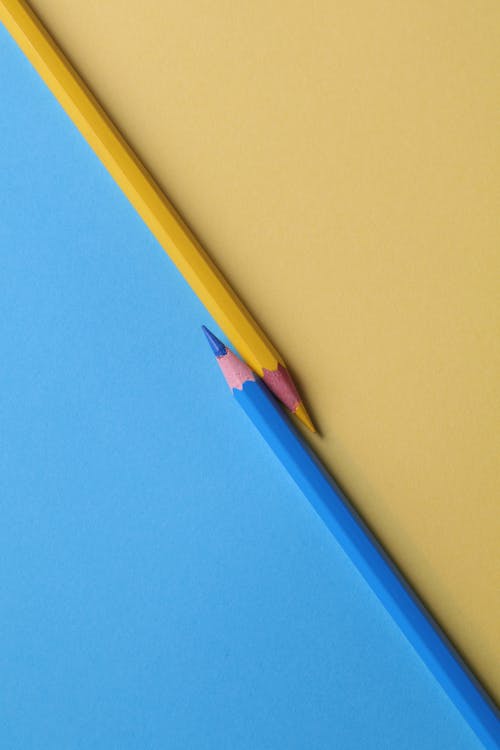
After post-processing: Notice the enhanced contrast, subtle vignetting, and optimized color balance
Advanced Model Selection
Different AI models excel at different tasks:
- Stable Diffusion 2.0+: Improved overall quality and consistency compared to 1.x versions
- Realistic Vision models: Excellent for photorealistic portraits and scenes
- Dreamshaper: Versatile for creative and imaginative content
- SDXL models: Superior at handling complex prompts and larger resolutions
- Fine-tuned specialty models: Consider models optimized specifically for your content type (landscapes, portraits, abstract). This can save significant time when developing a series of related images.
When working with specialized content, research which models the community recommends for your specific use case rather than defaulting to the most recent version.
Different AI models produce distinctly different results even with identical prompts
Future Trends in AI Image Optimization
As AI image generation evolves, several emerging techniques show promise:
- Motion integration: Combining still image generation with animation capabilities
- Multi-view consistency: Generating multiple angles of the same subject while maintaining coherence
- Advanced composition control: More precise guidance systems for element placement and relationships
- Material and lighting systems: Physically-based rendering approaches for more realistic textures and illumination
- Real-time feedback loops: Interactive systems that allow on-the-fly adjustments during generation
Staying informed about model updates and new techniques will help you continue to refine your optimization strategies.
The future of AI image generation will likely include more interactive, dynamic, and physically accurate rendering systems
Conclusion
Optimizing AI-generated images isn't just about writing better prompts—it's about developing a comprehensive workflow that combines thoughtful prompt engineering, parameter tuning, and strategic post-processing.
The most successful AI artists develop a systematic approach to image creation, constantly refining their techniques through experimentation and careful analysis of results. By understanding the specific capabilities and limitations of your chosen AI models, you can push beyond basic generations to create truly exceptional visual content.
Remember that optimization is highly subjective and depends on your specific goals. What works for photorealism might not be ideal for stylized illustrations. Develop your optimization strategies based on your unique artistic vision and the specific requirements of each project.
As AI technology continues to advance at a rapid pace, the techniques that work today may evolve tomorrow. The most valuable skill is developing an experimental mindset—always testing, learning, and adapting your approach to leverage the latest capabilities these remarkable tools have to offer.
The art of AI image optimization is a blend of technical knowledge, artistic vision, and continuous experimentation